Earnings Call API’s and AI: Combining Forces for Advanced Financial Analysis
Investing is sort of a game of what to do, and when to do it - and good information quickly can make the difference between a good.- or a bad decision at the end of the day. Earnings calls provide insights into company performance, expectations for the future, how management perceives the company's trajectory over the long term, and so on.
Historically, this has been a very effort, but sync with Earnings Call API’s and Artificial Intelligence (AI) is reinventing this domain. This compilation delivers invested analysts and monetary institutions' adroit applications for forward sophisticated capabilities of fiscal analysis.
How earnings call API’s tackle the issue
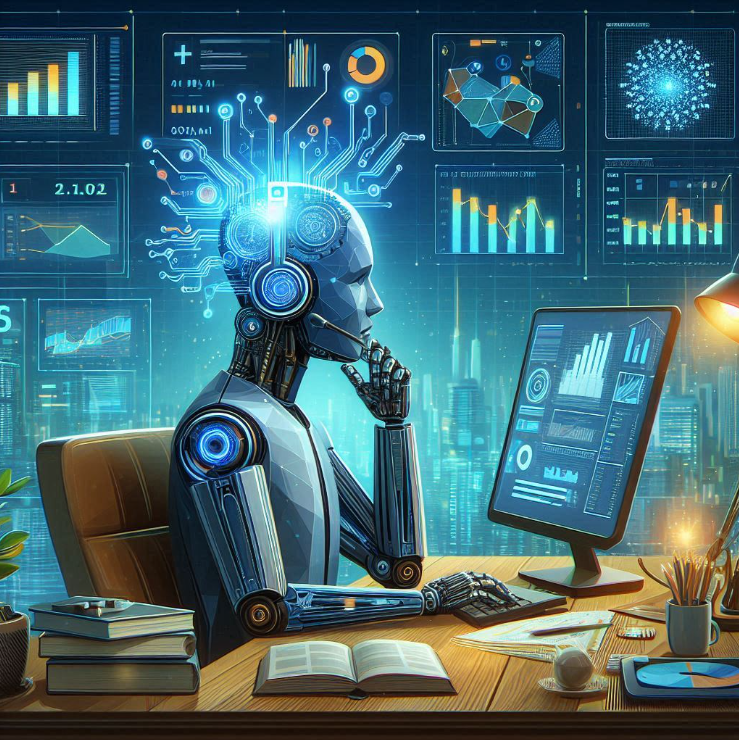
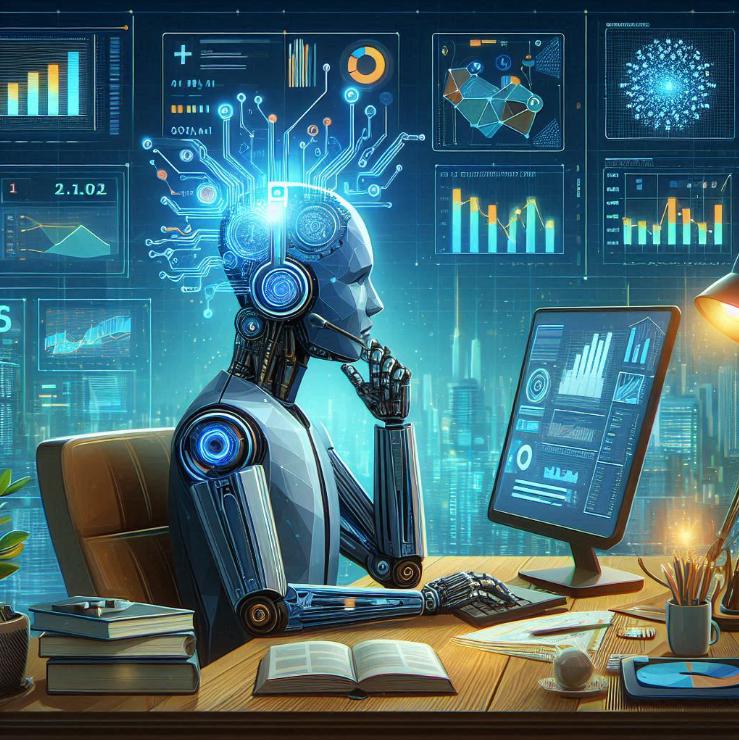
Earnings Call API’s offer an explosion of such information that can be programmatically accessed. These API’s typically offer:
Live Transcripts: Instant transcripts upon completion.
Historical Data: Past earnings call transcripts
Metadata: Contextual information like speaker identification, call dates, and financial periods.
Search: Search the transcript for specific keywords or over the entire transcription base.
API’s from AlphaSense, Seeking Alpha, Refinitiv, and others make it straightforward to incorporate this functionality into existing financial tools and platforms, enhancing the ability to automate earnings call data collection and analysis.
The Power of AI in Financial Analysis
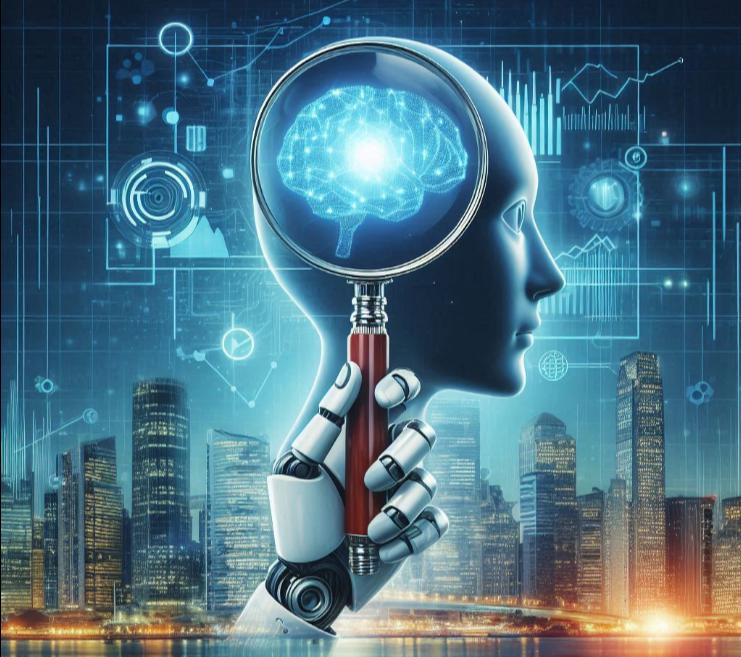
Machine learning, natural language processing (NLP), and other advanced techniques help you to process and interpret these oceans of data, for most financials, making use of AI for financial analysis. AI can be applied to:
Understand the Mood: Based on the statement from all the execs of FabFurnish, analyze the confidence for the market & the sentiment of the investor.
Trend Detection: Identify prominent themes and trends from earning calls over time.
Anomaly Detection: Unearth abnormal patterns or inconsistencies in financial conversations, possibly pointing toward problems or openings.
Predictive Analytics: Estimate how well you should expect the program to perform with historical data and current discussions.
To detect sentiment in webcasts and translate comments regarding certain events, NLP (natural language processing) tools such as IBM Watson, Google Cloud NLP, or custom AI models are required to be able to parse earnings calls for marketable insights.
Combining Earnings Call API’s and AI
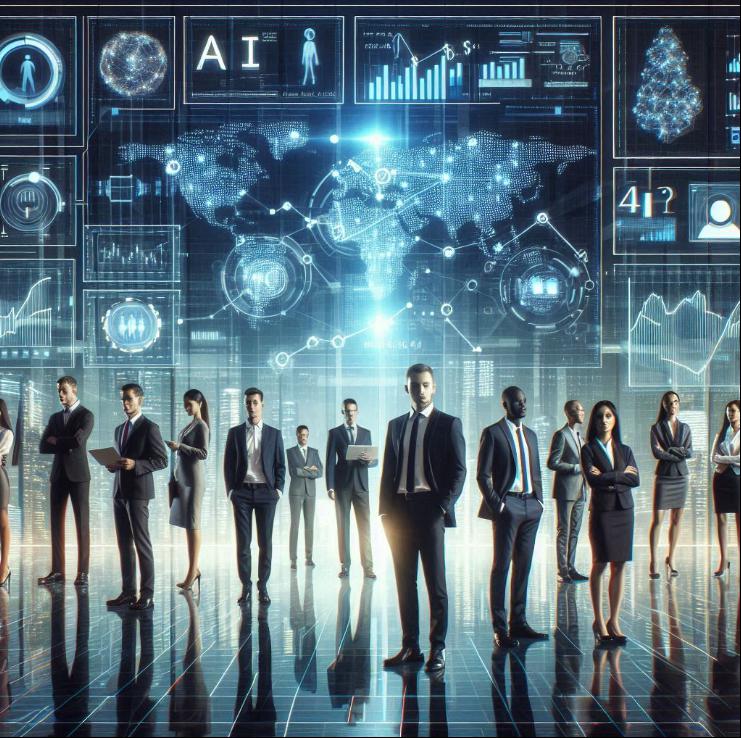
Earnings Call API’s along with AI, when combined, provide a two-sided coin to financial analysis. Here’s how:
Automated Data Collection and Processing: API’s delve out raw data on the fly, the AI in turn processes this data and isolates meaningful insights.
Better Decision Making: AI models comb through enriched data to pattern trends, sentiment, and anomalies, providing analysts with a richer insight into a business's performance.
Coming to Scalability: this combination can be used to analyze Big Data from a large number of industries and companies quite impossible with manual labor.
Performance and Speed: Real-time data extraction coupled with instantaneous analysis allows for faster responses to market shifts.
A financial analyst, for instance, can leverage an API to scrape from a site like Apple the most recent earnings call transcript. This transcript can then be analyzed by an AI model to identify sentiment, sentiment polarity, themes, and risks. For investment decisions - or strengthening advisory portfolios - an analysis like the one performed here on Twitter would have helped guide the way.
Case Studies and Applications
This powerful combination is already being used by multiple financial institutions and investment firms:
For Hedge Funds: The AI could provide sentiment and trend analysis from earnings calls for investment purposes.
Analyst research: Automate the review and summarization process of earnings calls, saving time, and increasing accuracy
Corporate finance teams: To track competition performance and market sentiment for strategic decision-making.
For example, a hedge fund would use an Earnings Call API to scrape data from hundreds of earnings calls every quarter. This data is processed by an AI system to identify sentiment trends in various industries, and to inform the fund's investment strategy.
Future Outlook
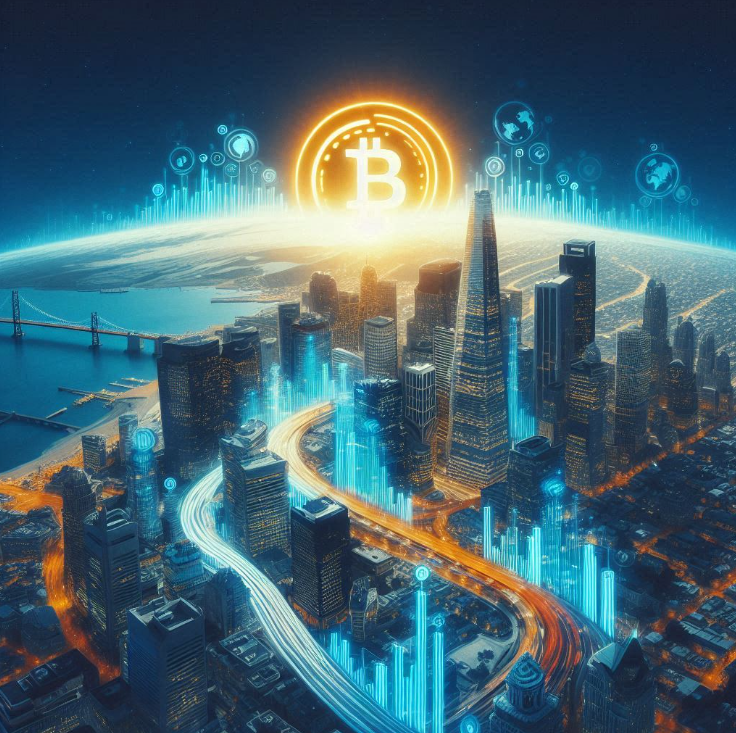
Next up is the coupling of Earnings Call API’s and AI, which promises a revolution in financial analysis. Further on, as AI develops itself, then we shall await more cutting-edge analyzing functions that can read in-between all the lines like :
Contextual Read: Machines to understand the context of financial chats including financial industry-specific vernacular and context.
Follow-On Analysis Tools: These are the platforms that can take the AI individual data, name field, and follow-on and ask follow-on drill down on those ideas that Burbank sees as interesting.
Integration with Other Data Sources: Integration of earnings call data with other financial data like market and economic indicators to generate in-depth analysis.
Conclusion
The use of Earnings Call API’s along with AI is a step towards a more advanced financial analysis. Investors and analysts alike can make more informed choices and be one step ahead in the ever-evolving financial landscape while using next-generation solutions for data collection that utilize sophisticated analysis mechanisms to synthesize information with greater insights. With advancements in this technology, its positive impact on financial analysis will do nothing but increase in the years to come, meaning tremendous potential for change and improvement.
For more info and detailed analysis of companies, and earnings call insights, check out our app or visit our website!